With artificial intelligence (AI) implementation, results may only be seen in the long term but why is that normal and what are some of the challenges organisations may face and some ways to overcome them?
Customers may not see what’s behind the scenes as what we do is embed AI into the solutions and business processes, but the importance is how AI is propelling end-to-end business processes. The current state of AI is enhancing the capabilities of organisations by adding to the efficiency and productivity of their business model.
https://www.youtube.com/watch?v=voAohI72LX4
In the enterprise, there is one way to organise the AI initiatives and that is into three distinct buckets. First, AI-assisted analytics refers to the ability to analyse thousands of variables to determine patterns which provides decision makers useful insights for agile decision-making that are essential in today’s highly volatile world. Second, with AI augmentation, it is all about learning to supplement human operators to work more efficiently and with increased productivity. These could include robotic process automation and invoice processing. Finally, rethinking the end-to-end business process and leveraging AI/Machine Learning to completely change the incumbent business models is part of AI-driven business process transformations. It is in a budding stage, but we can already see some emerging signs in the digital supply chain, business networks and some industries that are asset intensive.
Overcoming Challenges One Strategy at a Time
Transformation is not without challenges and one could be the lack of a clear and standardized strategy to guide the process. It would be ideal to have a clear definition of a problem statement and an achievable outcome. But an issue is that in most of these cases, there’s a lot of data and that comes with an uncertainty as to what the problem to be solved is. Of course, there are no fixed solutions to problems. In the long term, the key performance indicator for us is always adoption. When one looks at business processes from an endpoint, it is also easier to measure the productivity and efficiency of each process and once measured, that can provide some numbers to show returns.
With every project, there is a need for a sponsor in the company who recognises in the long term benefits and who is willing to invest in the project even when results may come in the mid-long term. AI projects sometimes don’t always succeed in a certain timeframe, but in most organisations quick results are usually preferred and switching to the next big idea is likely without a long-term sponsor. With AI, it is a long journey so there is a need to keep chipping away at the idea for weeks or months. An important aspect to remember about AI is that the return on investment gets better over time as the underlying AI models adapt and improve. Therefore, the ability to stay on course is a very important factor in determining the success of AI projects.
A lack of easy data integration with machine learning systems can make it difficult for businesses to switch to integrating AI swiftly. Project costs increase as interoperability decreases, especially with maintenance fees. There can also be an increase in data silos occurring and the likelihood of poor data precision which could cause cascading quality and model accuracy issues along the line. Companies can be comfortable with existing business models and processes which means end-to-end transformation for to harness the full power of new technologies such as AI in order to disrupt the way they do business is not a priority. In fact, AI loves big data. While thinking about an AI strategy, companies need to also think about their overall data strategy – what should be kept, what should be leveraged, and what data can be archived.
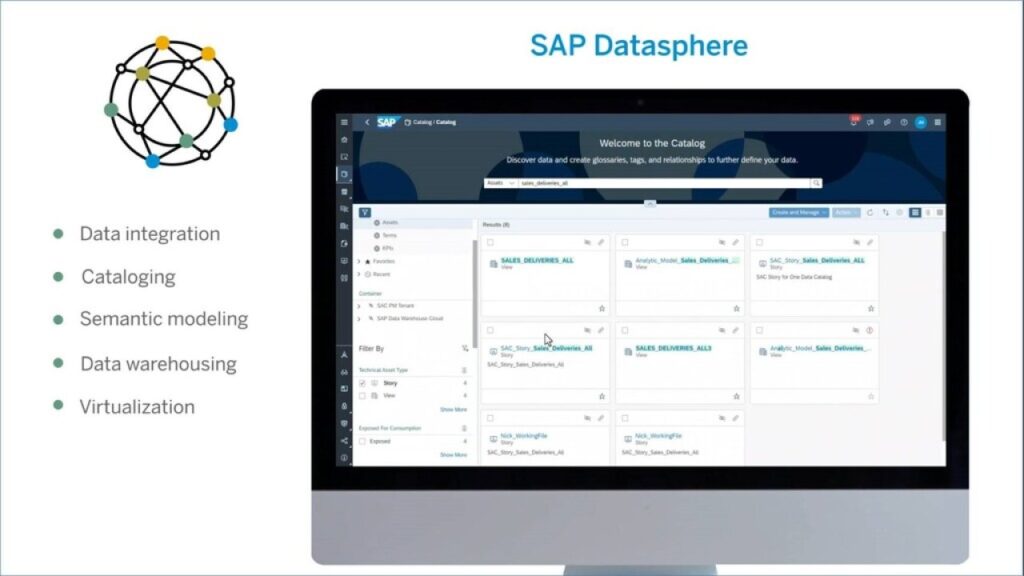
There’s always been a challenge to find qualified developers because the skill sets are different and are in high demand. For example, developers of AI/Machine Learning need to be highly skilled in mathematics, statistics and data science as well as be competent in development/data architecture to be able to bring projects to life. Contrasting this to 20 years ago, a developer was more likely defined by the programming languages they know.
It’s a very competitive market and has been the case for the last decade in this space. In Singapore, the government is working with a number of local universities to embed AI, machine learning, and data science in a lot of the courses being run so there’s a number being pumped into the industry these days which is a great start to overcoming the current shortages.
Where Is This All Leading Towards in the Next Few Years?
These challenges are not independent of each other and are not isolated among different organisations. Implementing AI requires different skill sets and knowledge compared to computer engineers/coders/developers, it also requires an understanding of data science and mathematics. With that in mind, there is a need to recognise that both public and private partnerships are important to foster AI skill sets for a future-ready workforce.
With the collaborations between organisations and local universities’ computer science courses such as the National University of Singapore and National Technological University, we are working to ensure that the future workforce is well-equipped with the necessary skillsets through industry upskilling and hiring of STEM talents as the gateway for product engineering in Asia Pacific and Japan.
According to IDC, global spending on AI technology will likely reach US$500 billion in 2023. This shows that it’s even more important than ever to build a technology strategy, incorporating AI and Machine learning, and leveraging the right software partners who can stay the course in the next three to five years with you.
Manik Saha is managing director of SAP Labs Singapore.